Data Governance Concerns of Agentic AI
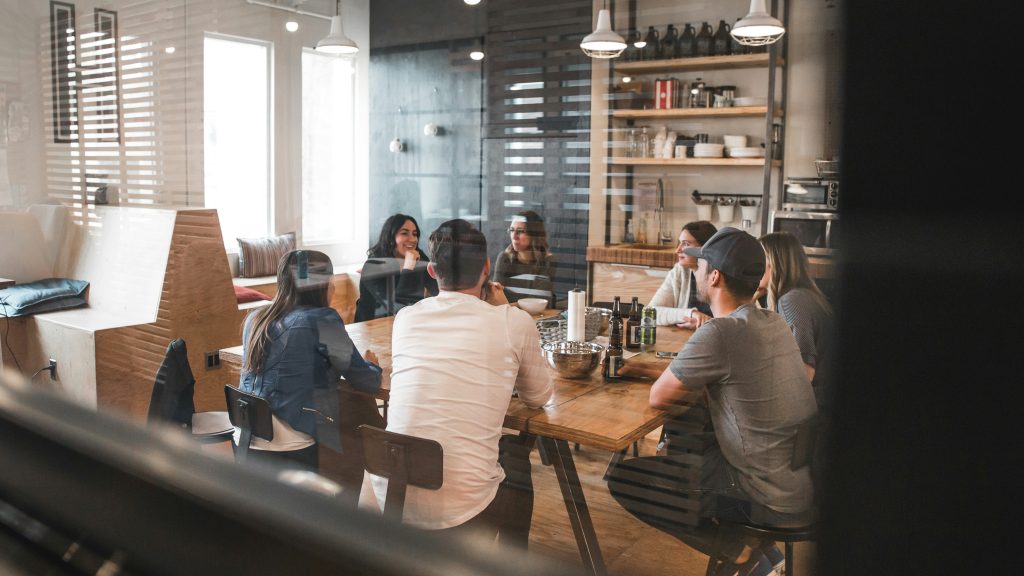
Agentic AI, characterized by autonomous decision-making capabilities, is revolutionizing industries by automating complex tasks and enhancing operational efficiency. However, this autonomy introduces significant data governance challenges that organizations must address to ensure ethical and effective AI deployment.
Understanding Agentic AI
Agentic AI refers to systems capable of setting their own goals, making complex decisions, and adapting to changing environments with minimal human intervention. Unlike traditional AI, which requires explicit inputs and produces predetermined outputs, agentic AI can receive instructions, create plans, and utilize tools to complete tasks, resulting in dynamic outputs.
Data Governance Concerns in Agentic AI
- Data Privacy and Security: Agentic AI systems often process vast amounts of sensitive data, raising concerns about unauthorized access and potential breaches. Ensuring robust data protection measures is crucial to maintain user trust and comply with regulations.
- Bias and Fairness: The quality of AI outputs heavily depends on the data used for training. Biased or unrepresentative data can lead to discriminatory outcomes, making it essential to implement rigorous data governance policies that dictate how AI agents collect, store, and use data.
- Transparency and Accountability: The autonomous nature of agentic AI can obscure decision-making processes, complicating accountability. Organizations must establish clear documentation and traceability protocols to ensure every decision made by the AI is understandable and justifiable.
- Regulatory Compliance: As AI technologies evolve, so do the regulations governing them. Organizations must stay abreast of legal requirements to ensure their AI systems comply with current laws and standards, thereby avoiding legal repercussions.
Best Practices for Data Governance in Agentic AI
- Implement Robust Data Management Frameworks: Develop comprehensive data governance frameworks that encompass data quality, privacy, and security to mitigate risks associated with agentic AI.
- Enhance Transparency: Utilize explainable AI techniques and conduct regular audits to build user trust and ensure the integrity of AI systems.
- Continuous Monitoring and Adaptation: Regularly update governance frameworks to align with evolving regulations and technological advancements, ensuring ongoing compliance and risk management.
Conclusion
While agentic AI offers transformative potential, it also presents complex data governance challenges. By proactively addressing these concerns through robust data management, transparency, and compliance strategies, organizations can harness the benefits of agentic AI responsibly and ethically.
For organizations seeking to assess and enhance their data governance practices, a Data Maturity Assessment can provide valuable insights into current capabilities and areas for improvement. Engaging with experts in data strategy and governance can further support the development of effective frameworks tailored to organizational needs.